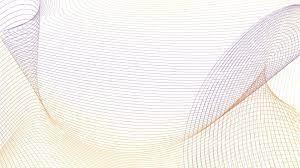
27 May 2024
https://www.itu.int/hub/2024/05/ai-watermarking-a-watershed-for-multimed...
Misinformation, deepfakes, and systemic biases have become a constant risk in business, academia, politics, and ordinary social interactions.
The growing proliferation of audio, video, image, and text content generated by artificial intelligence (AI) has unfortunately started blurring the line between what is real and what is fake.
Fortunately, international technical standards could soon help to make generative AI more trustworthy.
One proposed measure is “AI watermarking,” which should help to identify AI-generated multimedia works – and expose unauthorized deepfakes.
AI watermarking involves embedding markers into multimedia content for it to be accurately identified as AI-generated.
The technology is composed of two parts: the watermark-embedding algorithm and the watermark-detection algorithm. This combination creates a unique, identifiable signature – invisible to humans but detectable through algorithms and traceable back to the original AI content-generation model.
Watermarks are created during the model-training phase by teaching the model to embed a specific signal or marker in the content it generates. Then, after the AI model is deployed, specialised algorithms can detect the presence of that embedded “watermark.”
Alongside identifying content as AI-generated, well-designed watermarking should also make clear the provenance of such content.
For multimedia authenticity initiatives, AI watermarking offers important benefits, including:
- Authentication and validation: AI watermarking can provide a reliable method for authenticating and validating digital files. This is crucial in an era where deepfake videos, manipulated images, and deceptive media are prevalent.
- Tamper-evident records: The use of blockchain and public key infrastructure can help ensure that any attempts to alter or manipulate content are readily detectable.
- Trust and confidence: Content creators, distributors, and consumers can be assured of the authenticity of the media they encounter. This is crucial to combat misinformation and counterfeiting, as well as enhancing overall understanding of content provenance.
International standards needed
Various watermarking techniques are already on the market for use with AI-generated audio, images, video, and text.
Currently, however, the embedded markers can be modified and removed.
Questions also arise about who should provide AI detection tools and whether such tools should catch all AI-generated content or just the output of a certain model.
These are governance issues with implications for trust in AI systems.
Today’s watermarking techniques are yet not standardized, and a watermark generated by one technology may be unreadable or even invisible to a system based on a different technology.
Without standardized access to detection tools, checking if content is AI-generated becomes a costly, inefficient, and ad hoc process. In effect, it involves trying all available AI detection tools one at a time and still not being sure if some content is AI-generated.
The proliferation of generative AI necessitates a public registry of watermarked models, along with universal detection tools. Until then, ethical AI users must query each company’s watermarking service ad hoc to check if a piece of content is watermarked.
Open-source generative AI software tools raise additional important questions to maintain the integrity of AI watermarks.
While users are free to modify open-source software, a watermark should be an integral part of an AI model that cannot be modified or removed.
Open-source watermarking schemes present other challenges, such as the need for trusted custody of private information and potential for open-source schemes to be circumvented.
Further research is needed on watermarking without risk of watermarks be modified or removed as well as the pros and cons of making watermarking schemes publicly available.
Watermarking all training data could be attractive if the resulting generative AI tools are open sourced, amid concerns that watermarking processes could otherwise be removed from open-source software.
Initiatives for AI authenticity
The Coalition for Content Provenance and Authenticity (C2PA) – a collaborative initiative by leading firms including Adobe, Intel, Microsoft, and Sony – aims to establish standards for verifying the authenticity of audio-visual content. Among other technologies, C2PA is promoting is provenance data – invisible metadata embedded in digital content to identify the creator and verify the content’s authenticity.
Blockchain technology is immutable, making it ideal to record provenance. Once digital assets are recorded on blockchain, their ownership history is permanently and publicly recorded.
The Ethereum Improvement Proposal project calls for adding C2PA consent data, such as the Content Consent for AI/ML Data Mining, to on-chain metadata, using blockchain technology to provide additional security and transparency.
During the AI for Good Global Summit, a full-day workshop dives into multimedia authenticity issues, with a focus on international standards, the use of watermarking technology, enhanced security protocols, and cybersecurity awareness.
The free workshop, open to anyone interested, takes place on 31 May in Geneva, Switzerland, and online.
The session aims to unite leading minds and experts to discuss recent research findings and ongoing standardization efforts, aiming to create a collaborative platform to collectively address current gaps. It also aims to develop recommendations for practical actions and encourage further investment in this field.
Register for the workshop here: Detecting deepfakes and Generative AI: Standards for AI watermarking and multimedia authenticity.
Learn more about the AI for Good Global Summit.
In another blog post, Alessandra Sala delves into how AI-generated multimedia content has blurred the line between real and fake.
Header image credit: Adobe Stock/AI generated